Association Rule Mining
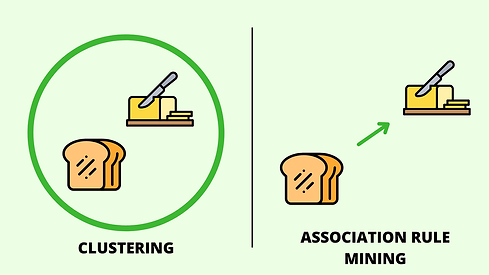
Association Rule Mining (ARM) is a technique used to uncover interesting relationships between variables in large datasets, often applied in market basket analysis and text analysis. ARM identifies patterns in words that frequently appear together. The key measures in ARM are support, confidence, and lift. Support measures how frequently an item (two words) appears in the dataset. Confidence reflects the likelihood that the consequent appears when the antecedent is present. Lift measures how much more likely the consequent is to appear compared to random chance. Rules in ARM show where the antecedent leads to the consequent.
​
The Apriori algorithm identifies frequent itemsets using a minimum support threshold and generates association rules based on confidence and lift.
​
ARM will be used to uncover patterns in how gendered words co-occur within news articles. The Apriori algorithm will identify frequent word pairings that may reflect underlying narratives or biases in gender representation. Association rules will highlight common links between gendered terms and specific topics, roles, or descriptors. This analysis will provide a more detailed understanding of word-level relationships, complementing the broader thematic patterns identified through clustering.

Data Prep
The count vectorized gender articles were transformed into a binary format, where each word's presence was represented as a 1 and its absence as a 0. This ensured ease of use in the apriori algorithm.
Before
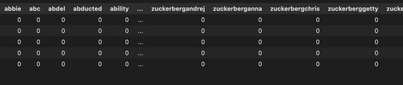
After
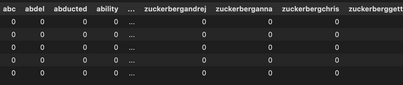
Results
The apriori algorithm and the rules were generated using a minimum support of 0.02 and a minimum threshold of 1.0. The top 15 rules reveal strong relationships between words frequently appearing together in news articles. In the support-based rules, the most common associations involve political figures, particularly "Trump," "Donald," and "President," which frequently occur together. This reinforces the findings that many of the January Business Insider articles focused on Trump's presidency.

The top 15 rules by confidence had 1.0, meaning that whenever the antecedent words appear, the consequent words are always present. All of the antecedents had "donald" or "trump," further emphasizing the dominance of Trump-related coverage in Business Insider.

The top 15 rules by lift strayed away from Trump-related discourse. Instead, it was heavily focused on Mark Zuckerberg and Meta and Elon Musk potentially showing insight into business-related articles. These results indicate that coverage of high-profile business figures is tightly clustered, with little room for deviation in the way they are discussed.​​
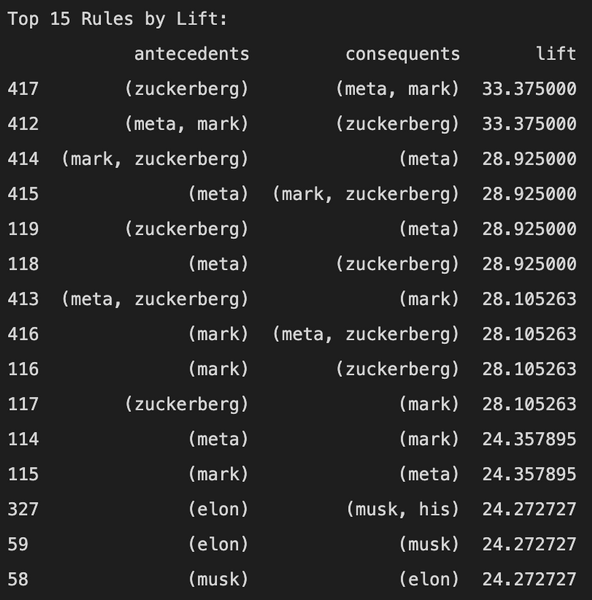
The ARM results highlight that the articles are heavily skewed toward politics and male business leaders, with predictable and repetitive patterns in word associations.
Gender-Related Networks
To analyze how gendered words appear in association rule mining, the rules extracted from the dataset were filtered to isolate those containing explicitly male- or female-gendered words. The networks below showcase association rules for men-related words ("he", "him", "his", "male", "man", "men") and women-related words ("she", "her", "female", "woman", "women"). Men-related words were strongly associated with Trump and Elon Must, reflecting their dominant presence in news coverage.
The women-related words were associated with "author," "courtesy" and "picture" potentially indicating editorial contexts. Moreover, a notable association with "kids" suggests that many gendered articles about women focus on caregiving roles and family-related responsibilities, reinforcing traditional gendered narratives in media representation.
​
This disparity in association patterns further emphasizes gendered narratives in media coverage, where men are more frequently linked with power and professional roles, while women are associated with caregiving and personal life.
ARM Network for Men-Related Words
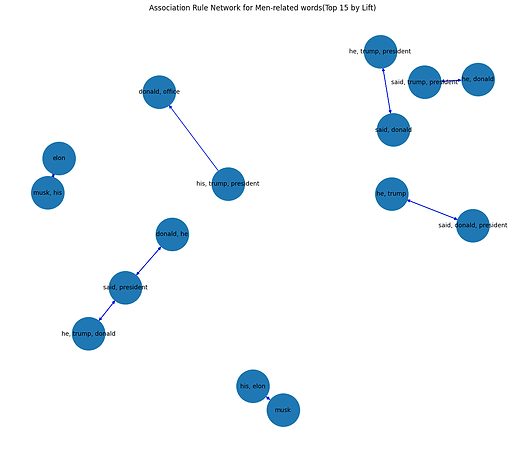
ARM Network for Women-Related Words
